The Impact of Generative AI on Work Productivity
Generative artificial intelligence (AI) has rapidly emerged as a potentially important workplace technology. In an earlier blog post, we discussed results from the first nationally representative U.S. survey of generative AI adoption, conducted in August 2024. We showed that 28% of all workers used generative AI at work to some degree. We ran our survey again in November 2024 and found that usage rates were fairly stable between August and November. In this blog post, we leverage a novel question in the November survey to provide an estimate of potential aggregate productivity gains from generative AI.For more details, see our updated working paper, “The Rapid Adoption of Generative AI,” Federal Reserve Bank of St. Louis Working Paper 2024-027C, revised February 2025.
How Much Work Is Assisted by Generative AI?
Measuring the impact of generative AI on work productivity requires looking beyond simple adoption metrics and assessing how intensively workers rely on the technology. By asking respondents how much they used this technology in the previous week or previous month, our survey elicits two measures of the intensity of generative AI usage: days per week and time per day when used. First, pooling our survey data from August and November 2024, we found that in the previous week, 9% of U.S. workers reported using it every workday and 14% for at least one but not every workday.
The figure below shows that among workers who reported using generative AI at least once in the previous month, nearly one-third (31.9%) spent an hour or more per workday using it, while another 47.0% used it between 15 and 59 minutes daily. The two intensity measures of generative AI use are positively correlated. For example, 52.0% of generative AI users employing this technology every workday also reported using it an hour or more daily, compared with only 7.3% of those who used it in the previous month but not the previous week.
Intensity of Generative AI Use for Work
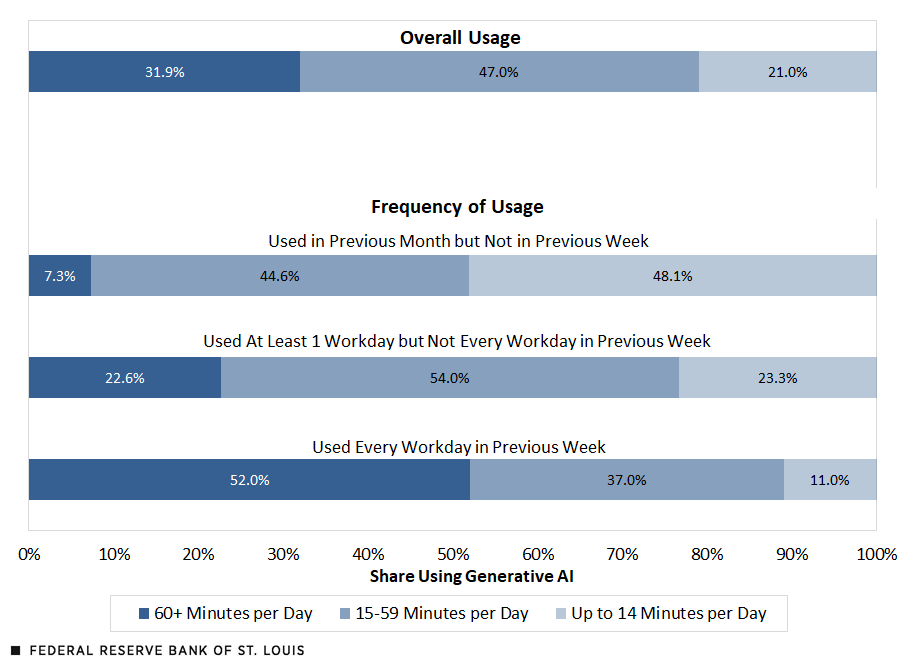
SOURCE: Bick, Blandin and Deming.
NOTE: “Overall” represents the distribution of usage among all survey respondents who reported utilizing this technology at work.
Using information on days worked and hours worked, we can construct bounds on the share of total work hours assisted by generative AI by combining data on usage intensity with data on days and hours worked in the previous week. Among workers who used generative AI in the previous week (21.8% of all workers), between 6.0% and 24.9% of all work hours were assisted by generative AI. This means that, on average, generative AI is not just an occasional tool for its users but also an integral part of their work routines. Among all workers, including those who used it only in the previous month and non-generative AI users, we found that between 1.3% and 5.4% of total work hours were assisted by generative AI.
Time Savings and Productivity Gains
To quantify the productivity benefits of generative AI, we asked November survey respondents how much additional time they would have needed to complete the same amount of work last week if they had not been able to this technology.In the survey, we asked: “You indicated that LAST WEEK you worked X hours and that you used Generative AI for your job. Now, imagine that LAST WEEK you did not have access to Generative AI. How many additional hours of work would you have needed to complete the same amount of work?” (X represents the value of the hours the respondent reported working last week.) The responses suggest that users save a meaningful amount of time.
The next figure shows that among workers who used generative AI in the previous week, 20.5% said that generative AI saved them four hours or more in that week, 20.1% reported three hours, 26.4% reported saving two hours, and 33.0% reported an hour or less. More-frequent users also reported greater time savings. Among workers who used generative AI every day in the previous week, 33.5% said it saved them four hours or more, compared with 11.5% of those who used it only one day in that week.
Time Savings by Frequency of Generative AI Use for Work in Previous Week
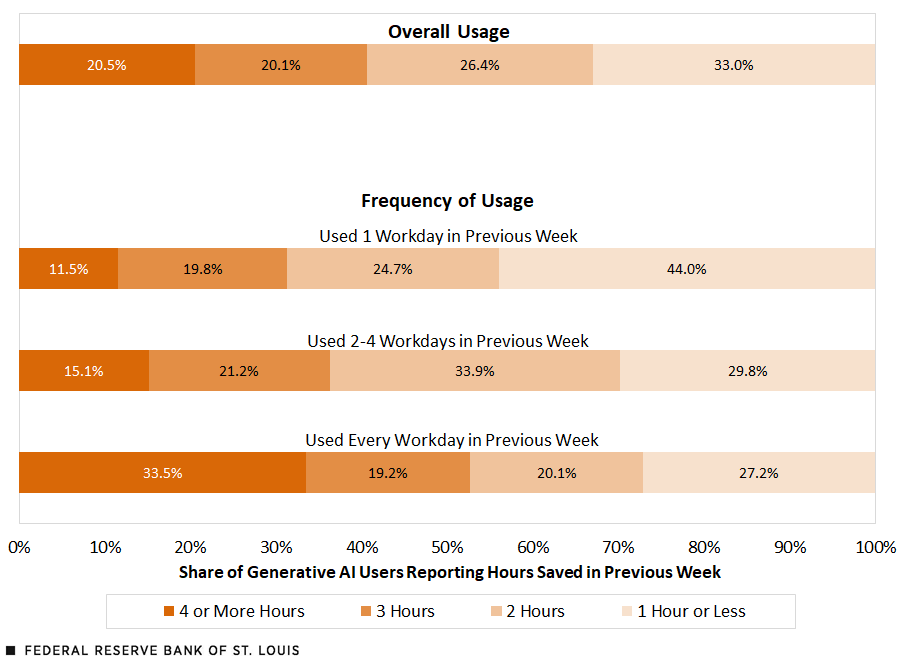
SOURCE: Bick, Blandin and Deming.
For each generative AI user, we computed the percentage of working hours saved as the ratio of time saved in the previous week to hours worked in that same week. We found an average time savings of 5.4% of work hours in the November 2024 survey. For an individual working 40 hours per week, saving 5.4% of work hours implies a time savings of 2.2 hours per week. When we factor in all workers, including nonusers, workers saved 1.4% of total hours because of generative AI.
Usage and Time Savings among Occupations and Industries
The next figure illustrates how generative AI-driven time savings vary with usage across occupations. Time savings and overall usage are highly correlated. Workers in the computer and mathematics occupation used generative AI in nearly 12% of their work hours, and they reported this saved them 2.5% of work time. By contrast, workers in personal service occupations used this technology in only 1.3% of their work hours, and it saved them only 0.4% of work time. The slope of the dashed regression line is 0.17, indicating that a 10 percentage point increase in the share of time spent using generative AI is associated with a 1.7 percentage point increase in the time saved as a share of hours worked.
Average Time Savings from Generative AI Usage: By Occupation
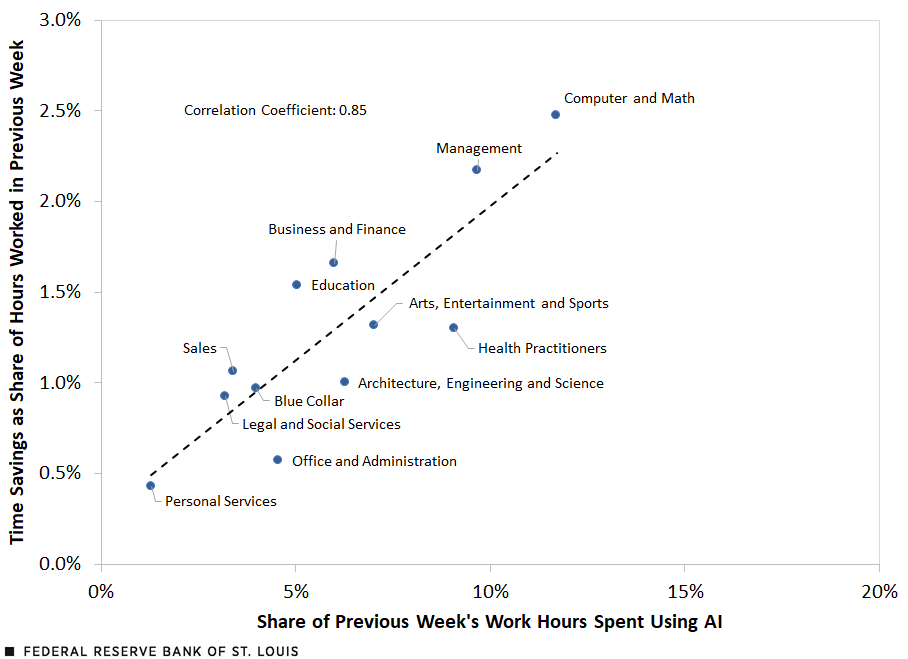
SOURCE: Bick, Blandin and Deming.
NOTE: Dashed line is a regression line.
The following figure shows that across industries, information services has both the largest share of work hours spent using generative AI (14.0%) and the highest time savings (2.6%). Leisure, accommodation, and other services has both the lowest share of work hours spent using generative AI (2.3%) and the lowest time savings (0.6%).
Time Savings from Generative AI Usage: By Industry
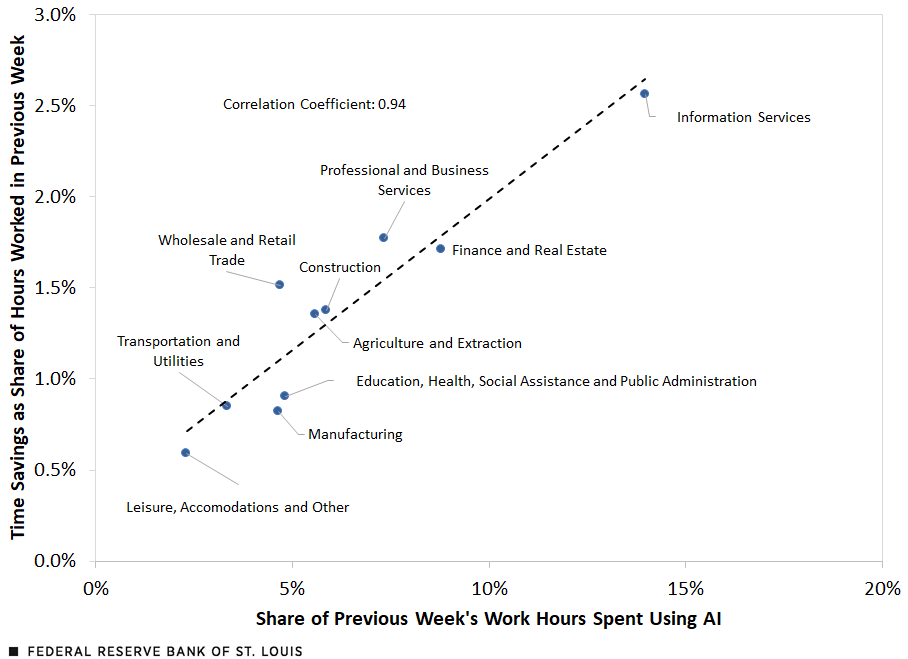
SOURCE: Bick, Blandin and Deming.
NOTE: Dashed line is a regression line.
In our working paper, we used a standard model of aggregate production and showed how we could use our data on hours worked, hourly wages and time savings from generative AI to provide a rough estimate of the aggregate productivity gain from that new technology. Together, the model and data imply that the self-reported time savings from generative AI translate to a 1.1% increase in aggregate productivity. Using our data on generative AI use, this estimate implies that, on average, workers are 33% more productive in each hour that they use generative AI. This estimate is in line with the average estimated productivity gain from several randomized experiments on generative AI usage.
Potential Gains May Not Yet Be Fully Captured in Measured Productivity
While we documented sizable time savings and a meaningful potential productivity gain, how these gains translate into measured aggregate productivity remains uncertain. If workers are now able to complete the same tasks in less time without their employers’ knowledge, they may take their time savings as on-the-job leisure, which would increase welfare but not productivity.
A 2024 working paper by Kathryn Bonney and others found that only 5.4% of firms had formally adopted generative AI as of February 2024. While firms’ adoption may increase over time, it still lags far behind, suggesting that worker adoption remains mostly informal. Thus, we emphasize that these potential productivity gains from generative AI may not immediately appear in productivity statistics, at least for now.
Future research should examine how firms adjust their expectations and work processes in response to widespread AI adoption. As more companies formally integrate AI into workflows, we may see these gains materialize more clearly in aggregate productivity measures. The extent to which AI reshapes the labor market—whether through job displacement, wage effects or skill development—remains an open and crucial question.
Notes
- For more details, see our updated working paper, “The Rapid Adoption of Generative AI,” Federal Reserve Bank of St. Louis Working Paper 2024-027C, revised February 2025.
- In the survey, we asked: “You indicated that LAST WEEK you worked X hours and that you used Generative AI for your job. Now, imagine that LAST WEEK you did not have access to Generative AI. How many additional hours of work would you have needed to complete the same amount of work?” (X represents the value of the hours the respondent reported working last week.)
Citation
Alexander Bick, Adam Blandin and David Deming, "The Impact of Generative AI on Work Productivity," St. Louis Fed On the Economy, Feb. 27, 2025.
This blog offers commentary, analysis and data from our economists and experts. Views expressed are not necessarily those of the St. Louis Fed or Federal Reserve System.
Email Us
All other blog-related questions